An vital attribute of main depressive dysfunction (MDD) is that the signs can range fairly a bit from affected person to affected person (Musliner et al. 2016). Moreover, therapy success is affected person particular, with 20-25% of the MDD sufferers liable to creating persistent melancholy (Penninx et al. 2011). Subsequently, latest analysis has sought to search out biomarkers that may assist information therapy choices and support our prediction of therapy outcomes (Gadad et al. 2018). The hope is that by tailoring the remedies to the sufferers, notably for sufferers at excessive threat, the remission fee might be improved.
It’s recognized that persistent melancholy is related to a selected set of signs, together with:
longer symptom period, elevated symptom severity and earlier age of onset; greater ranges of neuroticism and decrease ranges of extraversion and conscientiousness; and varied inflammatory markers, low ranges of vitamin D, metabolic syndrome and decrease cortisol awakening response (Habets et al. 2023)
Nonetheless, earlier research which have tried to foretell individual-level therapy response haven’t been very profitable. Subsequently, Habets et al. (2023) used multi-omics knowledge (lipid-metabolomics, proteomics, transcriptomics, and genetics), demographic, physiological and scientific knowledge together with a non-linear prediction technique to seize the complicated pathophysiology of MDD. The authors generated totally different prediction fashions and evaluated how nicely every mannequin predicts MDD remission after two years.
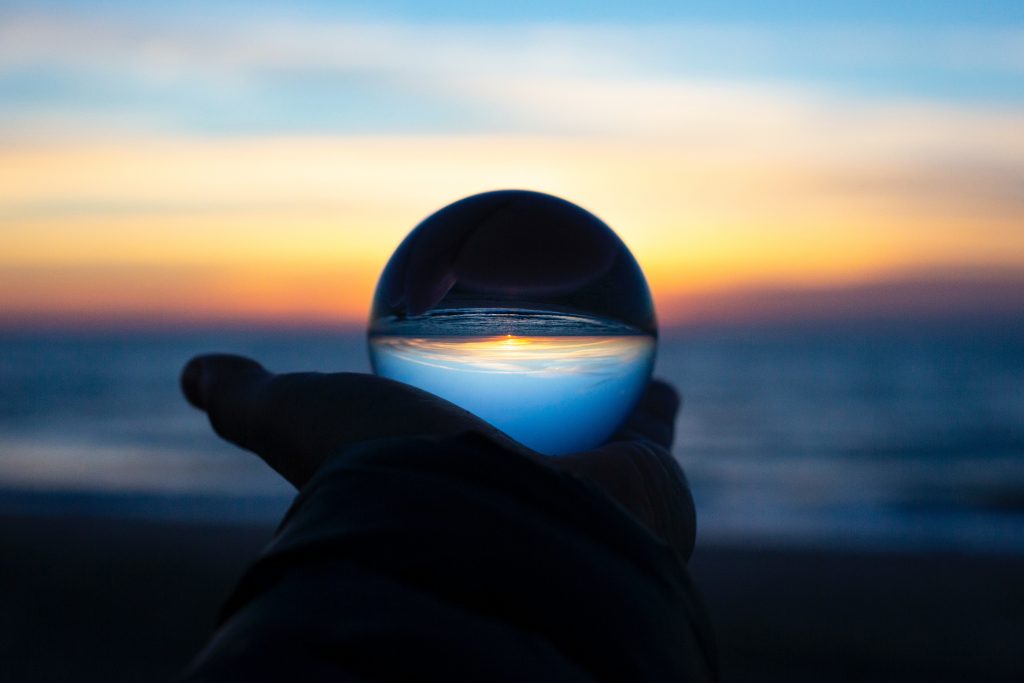
Melancholy signs and response to therapy differs vastly from affected person to affected person and fashions making an attempt to foretell therapy response haven’t been very profitable so far.
Strategies
804 individuals from the Netherlands Examine of Melancholy and Nervousness (NESDA) have been included. All individuals had a melancholy or dysthymia prognosis, assessed by the Composite Worldwide Diagnostic Interview (CIDI) within the 6 months previous to participation and so they have been assessed once more utilizing the identical software at a 2 12 months comply with up.
Along with routine scientific knowledge like depressive symptom severity, proteomics (out there for n = 611), lipid-focused metabolomics (n = 790), transcriptomics (n = 669) and genetic knowledge have been collected (n = 701). For each set of information, a prediction mannequin was educated individually with a non-linear method, known as XGBoost, utilizing cross validation. Because of this the mannequin was repeatedly educated on subsets of the info and evaluated on the left-out knowledge to evaluate how nicely the mannequin can generalise to unseen knowledge. Then, extra fashions have been educated utilizing a mix of the scientific knowledge and every of the omics datasets in addition to a utilizing a mix of all datasets. The efficiency of the prediction mannequin was assessed by the world underneath the receiver working attribute curve (AUROC) in a separate check set (20% of complete pattern). The AUROC is a measure how nicely you possibly can carry out the prediction, the place 0.5 is equal to guessing and 1 means a powerful prediction (i.e., excessive sensitivity and specificity).
To check the significance of every variable used by way of their predictive capability, SHAP evaluation was carried out on the proteomics and proteomics plus scientific knowledge fashions. Lastly, 4 scientific psychiatrists have been requested to foretell the chance of remission for 200 sufferers based mostly on both 10 or 17 scientific variables.
Outcomes
So, which set of information greatest predicted therapy response when utilized in isolation?
- All fashions had an accuracy stage above probability. Nonetheless, the mannequin based mostly solely on polygenic threat scores (PRS), a method used to calculate one’s genetic threat of getting a sure consequence, was biased in the direction of false unfavourable classifications. Because of this the mannequin tended to wrongly classify people who have been in remission as having melancholy. You may learn extra about polygenic threat scores on this latest Psychological Elf weblog on Tourette Syndrome (Palmer, 2023).
- The mannequin educated solely on proteomic knowledge had the very best AUROC of 0.67.
- The subsequent greatest mannequin was based mostly on 10 scientific variables (AUROC = 0.63). These included age, intercourse, years of training, and depressive symptom severity, as measured by the Stock of Depressive Symptomatology – Self-Report (IDS-SR) in a steady and categorical vogue, and 5 character dimensions.
- A mannequin based mostly on 63 scientific variables didn’t carry out any higher than the mannequin based mostly on 10 variables.
Mixture of omics and scientific knowledge
When scientific data was added to the omics datasets, the fashions all outperformed their respective particular person fashions, which included solely the omics knowledge. The mixture of scientific and proteomics knowledge had the very best AUROC of 0.78. This was additionally the one mixture of datasets the place the distinction in efficiency, when in comparison with the person datasets, was discovered to be vital (p<0.05). Curiously, all modalities collectively solely confirmed an AUROC of 0.70.
When utilizing linear prediction fashions, fashions based mostly solely on proteomics knowledge confirmed poor predictive efficiency. Furthermore, the addition of proteomics knowledge to scientific knowledge didn’t enhance the predictive efficiency.
Variable significance evaluation
In each fashions (proteomics alone and proteomics plus scientific knowledge), fibrinogen confirmed the very best variable significance. From the scientific knowledge, symptom severity at baseline was deemed most vital within the mannequin with scientific knowledge alone and proteomics plus scientific knowledge.
Protein-protein-interaction networks and pathway enrichment analyses have been additionally calculated individually for the proteomic variables that have been deemed vital for the prediction in each fashions. Networks concerned within the inflammatory response and lipid metabolism have been discovered. These networks confirmed that pathways associated to interleukin 10 signalling, chemokine signalling, ldl cholesterol esterification and reverse ldl cholesterol transport have been most vital for predicting remission consequence.
Clinician prediction of remission
For the needs of comparability, clinicians have been requested to foretell the remission standing for 200 sufferers based mostly on scientific knowledge. The clinicians’ rankings confirmed a low common accuracy of 0.51. Curiously, offering extra scientific variable data solely marginally elevated this prediction accuracy (0.55).
Each prediction fashions educated utilizing this similar scientific knowledge outperformed the human raters (AUROC of 0.63 and 0.65, respectively).
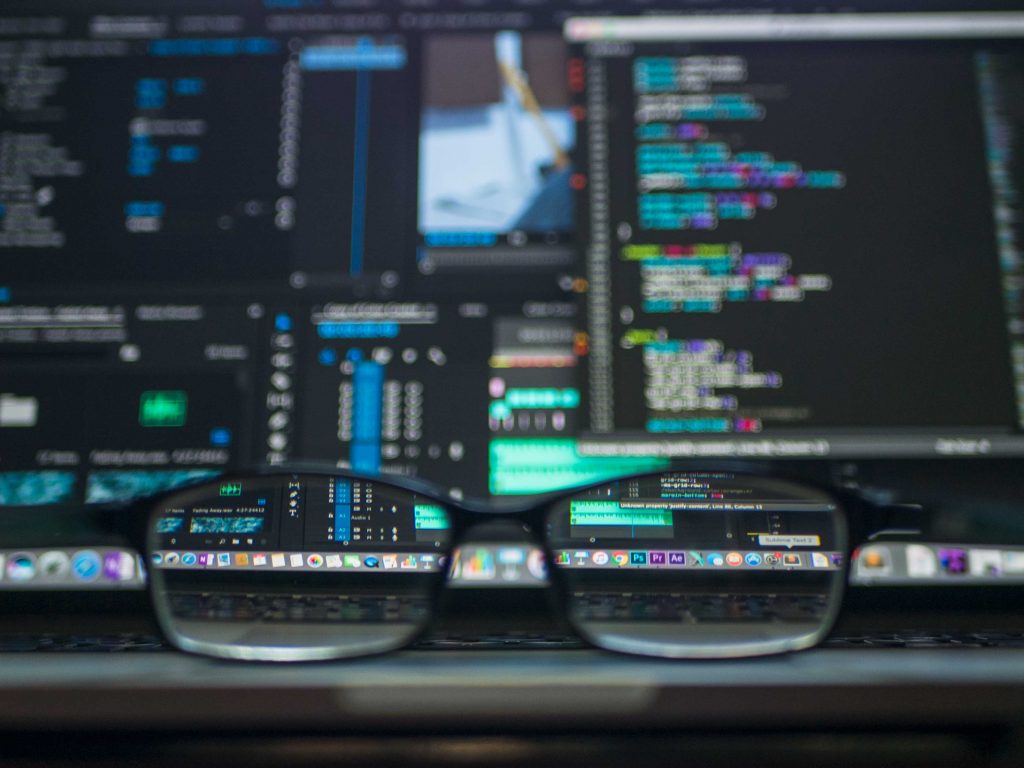
Prediction fashions utilizing each scientific and proteomic knowledge confirmed the very best efficiency. These fashions additionally each outperformed clinician remission predictions.
Conclusions
This examine confirmed that combining datasets from totally different domains and utilizing a non-linear mannequin can enhance prediction efficiency as in comparison with beforehand utilized easier approaches.
The authors conclude that:
this examine reveals that what’s predictive of remission of MDD inside 2 years is a mixed signature of symptom severity, character traits and immune and lipid metabolism associated proteins at baseline.
Although the balanced accuracy of 71% continues to be too low for scientific use, this mannequin however performs higher than predictions made by clinicians themselves. Subsequently, this examine might be seen as a place to begin, highlighting which knowledge sorts are most informative for machine studying fashions that ought to finally be examined in scientific trials.
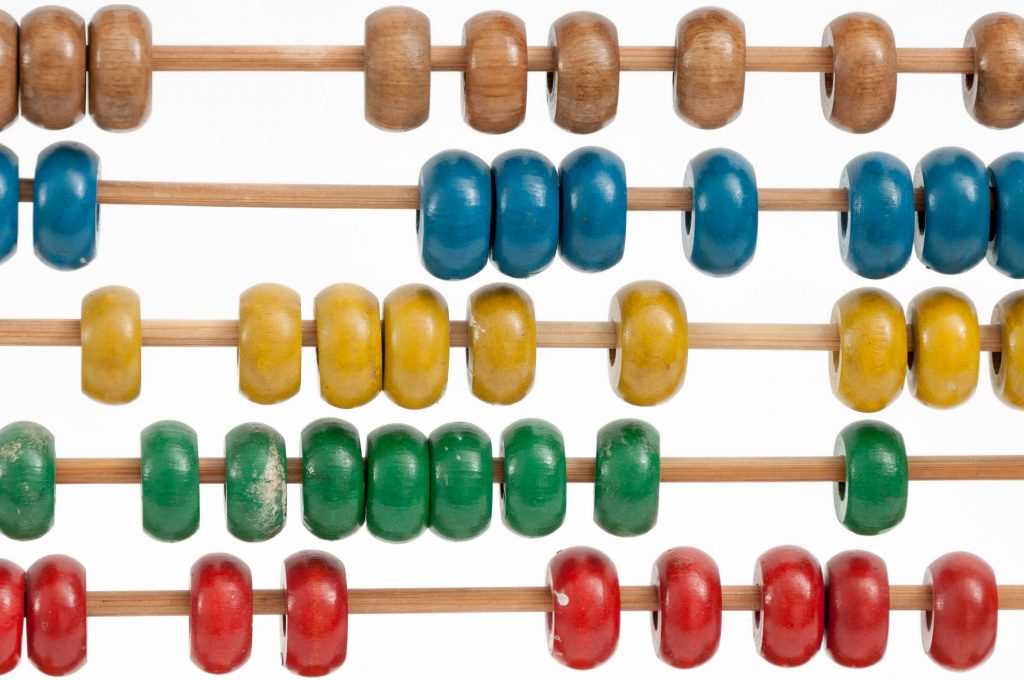
This examine means that symptom severity, character traits and proteins associated to immune and lipid metabolism can greatest predict melancholy remission after 2 years.
Strengths and limitations
Strengths
- The authors used a relatively massive dataset for which multi-omics knowledge was measured and the prediction modelling was arrange nicely with cross validation.
- The pre-processing of the info was performed in a way that ensured no data was leaked from the coaching to the check set.
- Moreover, they used a separate check set that was not a part of the cross validation for mannequin analysis.
- Lastly, they selected an extended sufficient comply with up timepoint of two years to permit for the analysis of melancholy remission in a significant method.
Limitations
- The mannequin was evaluated utilizing sufferers from the identical cohort as these included within the coaching set. This might inflate the mannequin efficiency and cut back its generalisability.
- Additionally, the imply accuracy of 71% continues to be too low for common scientific observe.
- As well as, the variety of predictor variables used should be diminished in order that they are often reliably and cost-effectively measured in a scientific laboratory.
- Lastly, crucial proteomic analyte, fibrinogen, was beneath the decrease restrict of detection in almost 70% of the samples. It is a common prevalence when utilizing the strategies utilized on this examine, nonetheless, one wants to stay cautious when decoding this discovering consequently.
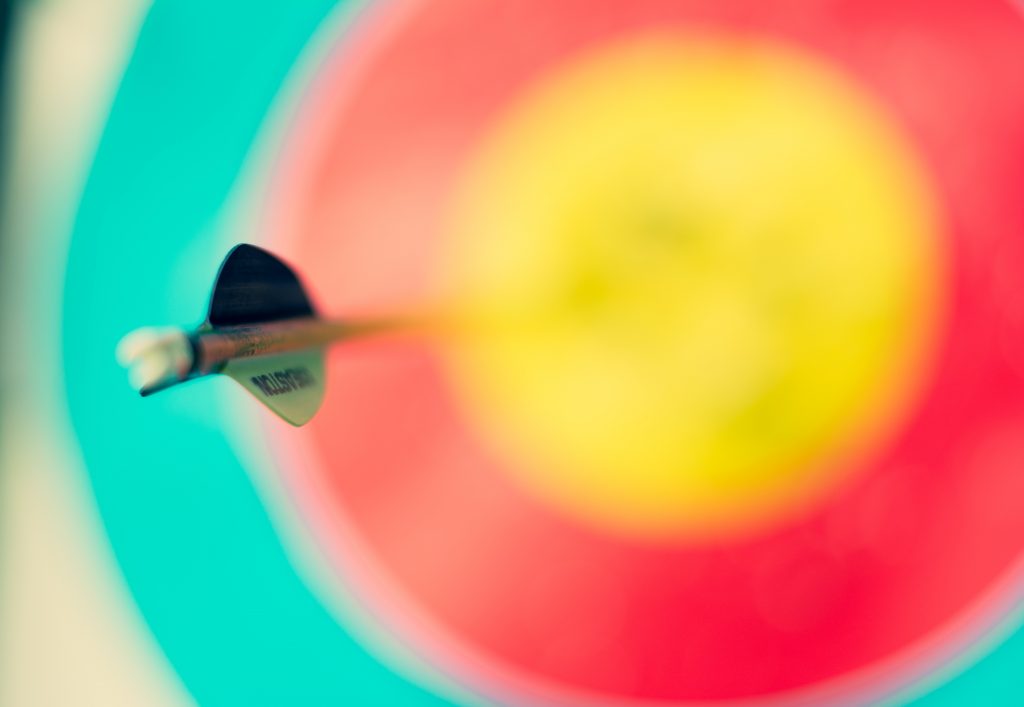
Whereas this examine was nicely performed and has addressed a number of limitations of earlier depression-focused prediction fashions, the general accuracy of the ultimate mannequin continues to be too low to contemplate its use usually scientific observe.
Implications for observe
It’s promising that this examine reveals that the addition of proteomics knowledge to scientific knowledge will increase the accuracy of the mannequin predictions for melancholy remission after two years. This reveals that it’s doable to search out biomarkers which can be associated to the situation.
For researchers, it’s attention-grabbing that the very best omics dataset was proteomics. Most frequently, transcriptomics or genomics knowledge are used as a result of they are often simply measured in a high-throughput vogue and are comparatively cost-effective. In distinction, proteomic measurements will not be as frequent. In immunopsychiatry research, typically the protein focus of only some immune markers are measured (e.g. interleukin (IL) 1 alpha, IL-6, tumour necrosis issue alpha or C-reactive protein). Habets et al. (2023) present proof that researchers may gain advantage from utilizing a extra common proteomics method.
For clinicians, this examine reveals that there’s worth in biomarker knowledge. The elevated accuracy of the predictions when proteomics have been added to the scientific knowledge is proof of this. This notably reigns true compared to the clinicians’ personal prediction rankings. Maybe the long run lies in utilizing machine studying fashions and multi-omics knowledge to assist practitioners of their remission consequence predictions and, finally, their therapy response predictions.
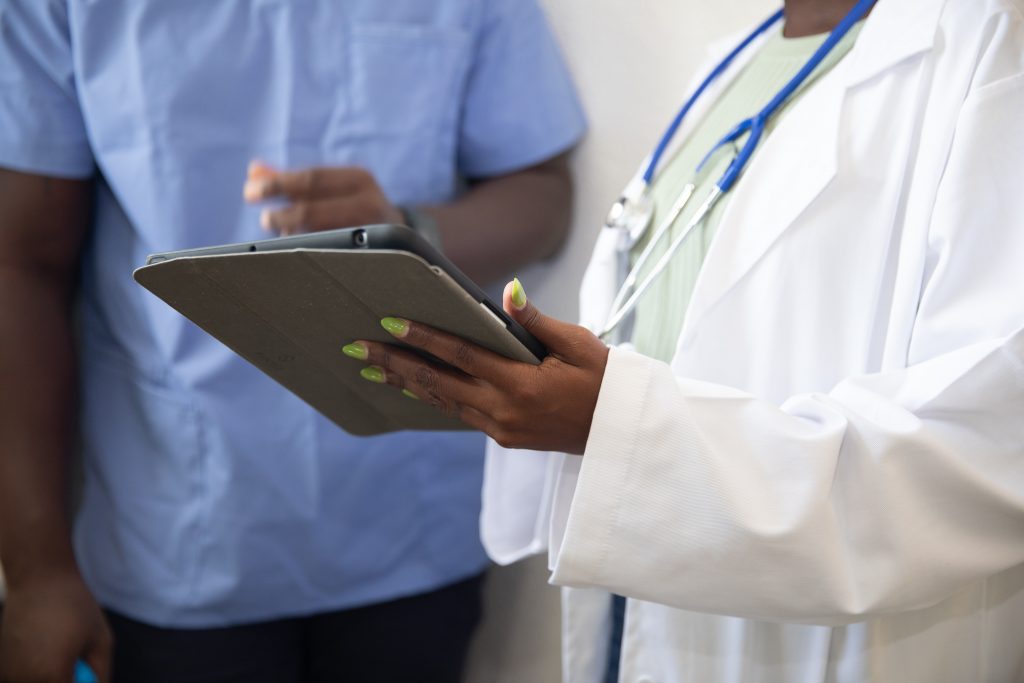
Machine studying fashions utilizing multi-omics knowledge may at some point assist clinicians of their predictions of remission consequence and therapy response in melancholy.
Assertion of pursuits
No conflicts to declare.
Hyperlinks
Main paper
Different references
Gadad, Bharathi S., Manish Okay. Jha, Andrew Czysz, Jennifer L. Furman, Taryn L. Mayes, Michael P. Emslie, and Madhukar H. Trivedi. 2018. “Peripheral Biomarkers of Main Melancholy and Antidepressant Therapy Response: Present Data and Future Outlooks.” Journal of Affective Problems, Are there Biomarkers for Temper Problems?, 233 (June): 3–14. https://doi.org/10.1016/j.jad.2017.07.001
Musliner, Katherine L., Trine Munk-Olsen, William W. Eaton, and Peter P. Zandi. 2016. “Heterogeneity in Lengthy-Time period Trajectories of Depressive Signs: Patterns, Predictors and Outcomes.” Journal of Affective Problems 192 (March): 199–211. https://doi.org/10.1016/j.jad.2015.12.030
Palmer, E. Genetic risk for Tourette Syndrome and related conditions. The Psychological Elf, 23 November 2023
Penninx, Brenda W. J. H., Willem A. Nolen, Femke Lamers, Frans G. Zitman, Johannes H. Smit, Philip Spinhoven, Pim Cuijpers, et al. 2011. “Two-Yr Course of Depressive and Nervousness Problems: Outcomes from the Netherlands Examine of Melancholy and Nervousness (NESDA).” Journal of Affective Problems 133 (1): 76–85. https://doi.org/10.1016/j.jad.2011.03.027